Innovative analytics
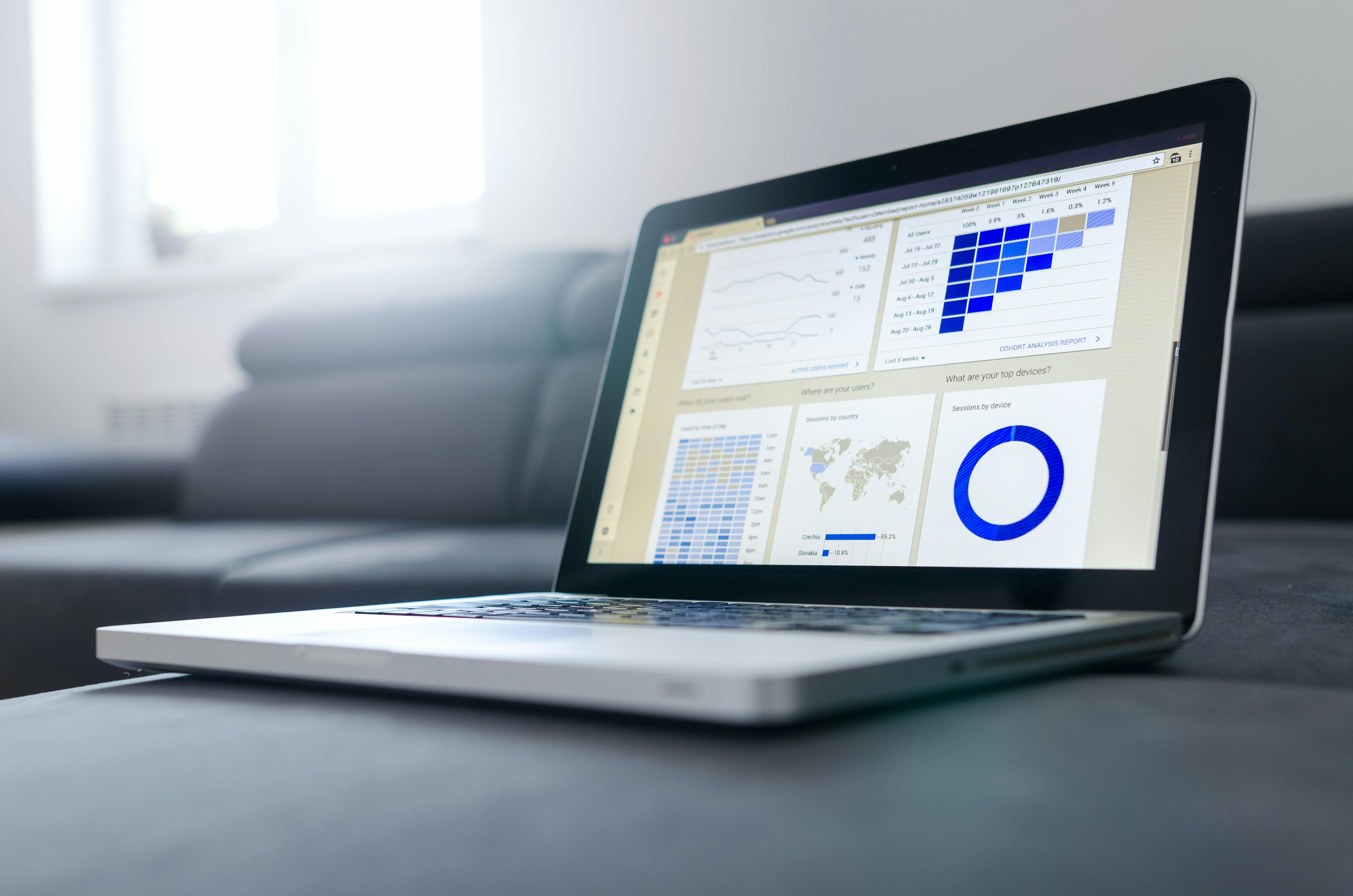
IET is an internationally recognised leader in learning analytics, with a rich history of researching, developing, and disseminating new approaches for the use of data in learning.
Through this workstream, IET uses its expertise to empower the successful use of data and learning analytics to improve the quality of teaching and learning and student outcomes. This is achieved through the development of new methodologies to enable leadership in evaluating and embedding predictive analytics in teaching practice, creating and establishing new ways to help disciplines at the OU understand and map their qualifications. It is also recognised for the role of supporting the OU to develop new innovative ways to evaluate teaching and learning interventions, as well as explore the potential of text analytics for summarising high volumes of student comments and providing data-rich student engagement to educators.
Learning Gains
The HEFCE/Office for Students Learning Gains project explored an Affective-Behaviour-Cognition (ABC) model of learning, to broaden the concept of learning gain, and - more importantly - to develop, test, implement, and evaluate a range of measurements for learning gains at each of the ABC level. The focus of the project was on cognitive learning gains. Five different approaches to measure cognitive learning gains (i.e., within module, from one module to the next one, within a qualification, across qualifications, across institutions) were used. Project findings indicated that although assessment scores could potentially be used as proxies for cognitive learning gains, a large proportion of these gains were not related to individual student ability, effort, and/or cognition. How teachers designed courses, marked and graded assessments substantially influenced the grade trajectories of students, which were disciplinary, and institutionally influenced and determined.
Student Pathways Analysis
The Open University offers a comprehensive curriculum for students, with flexibility in choice of modules, the order of study, and the length of time to complete a qualification. This flexibility frequently results in a large number of potential study paths to a qualification, each resulting in a different student experience. The Pathways Project aimed to find a way to analyse this complexity and thereby develop approaches to provide meaningful insight to practitioners and researchers. This research took a novel approach by employing a multi-model database, to analyse, map and visualise pathways taken by students (Edwards & Gaved, 2020a, 2020b). The overall aim is to improve our understanding of the student study experience, in order that we then improve it through developing our provision, the advice, and the guidance that we provide. The approach is being used to support several projects, funded by OU Scholarship Centres, with detailed qualification insight (Edwards 2021; Edwards 2022). The Pathways Project has also informed the EU funded project Erasmus+ Complex Trajectories project.
Predictive Learning Analytics
A key challenge of teaching at a distance is knowing when a learner may require additional support and assistance. Since 2017, we have worked closely with Associate Lecturers (ALs) from across faculties to study, evidence and enable adoption and use of the Early Alert Indicators dashboard presenting predictions as to who of the OU students are at risk of failing in their next assignment (Herodotou et al., 2020a). This work has resulted in module-focused studies and an emphasis on using predictive analytics to support students from Black, Asian and Ethnic minority students, in particular. In addition, we used randomised control trials to test specific teacher-supported interventions that could enable students at risk to succeed. During the 2022-23, we conducted a pan-university survey about the use of predictive learning analytics (n>300) with insight showing that interventions are needed to raise awareness of the dashboard across more ALs, organised professional development and briefing sessions involving 100 staff, submitted contributions to the OU Retention initiative, and supported successful intervention activities in the OU's Faculty of Business and Law.
Text Analytics of Student Feedback
Students’ responses to the open questions of end-of-module surveys provide invaluable insight into the students’ learning experience, however time and coordination propose challenges, to systematic analysis at an institutional level. Our work with Text Analytics of Student Comments (TASC) aimed to use automated text analytics methods to summarise and review the written feedback of our students. The text analytics techniques developed have provided insight for informing module changes (Brasher & Ullmann, 2020; Ullmann & Brasher, 2020), support for institutional analytics programmes (Ullmann et al., 2021) and compare and contrast the comments made by students from ethnic and minority backgrounds (Ullmann, 2021a). Funding from the university's Test and Learn project has supported IET in contributing insight and ideas to the development of a staff dashboard and sharing case studies about topic detection, sentiment analysis, qualification differences, and insights into high and low performing students (Ullmann & Rienties, 2021). Building on this work, IET is presently leading a new Test and Learn funded project to explore how Artificial Intelligence can support students to write reflectively. This should be written in the past as the project has now finished.
Innovative Methods
IET is at the forefront of exploring how new methodologies can support the evaluation of teaching and learning. For example, the QEI team have been interested in how non-randomised studies can improve the quality of teaching and learning evaluation. Statistical matching techniques, such as propensity score matching and others, can be used to create a fairer comparison between groups of students. IET has led the analysis component of a project investigating the impact of student performance of a new voluntary peer assessment activity on student performance (Ullmann, 2020), the benefits of phone call interventions (Kubiak and Ullmann, 2020), the effects of one-to-one tutoring for skills development (Ullmann, 2021b) and the implementation of a virtual microscope. Also, working with colleagues in the OU’s Faculty of Wellbeing, Education and Language Studies (WELS), the team have explored the potential of 360-degree spherical video. Outputs from this work include a framework for the use of spherical video in educational research (Cross, Wolfenden & Adinolfi, 2022a), framing the affordances and use of spherical 360-degree video in teacher professional development (TPD); Cross, Wolfenden & Adinolfi, 2022b) and providing recommendations as to how to improve the Virtual Microscope design to better support learners when engaging with it. This work highlights the importance of four key dimensions: open observation, critical distance, spatial presence, and investigative space.
Read more about QEI research below
Boroowa, A., & Herodotou, C. (2022). Learning analytics in open and distance higher education: The case of the Open University UK. In P. Prinsloo, S. Slade, & M. Khalil (Eds.), Learning analytics in open and distributed learning (pp. 46-62). SpringerBriefs in Education.
Brasher, A., & Ullmann, T.D. (2020). Text analytics to inform module changes: TASC pilot study 1. (QEI Report, Institute of Educational Technology). The Open University. Available on Scholarship Exchange.
Cross, S., Wolfenden, F., & Adinolfi, L. (2022a). Spherical 360-degree video recording and viewing in educational research: A case-study from India. In R. Sharpe, S. Bennett, & T. Varga-Atkins (Eds.), Handbook of digital education (pp. 211-223). Elgar Handbooks in Education.
Cross, S., Wolfenden, F., & Adinolfi, L. (2022b). Taking in the complete picture: Framing the use of 360-degree video for teacher education practice and research. Teaching and Teacher Education, 111, article no. 103597.
Edwards, C. (2022). FASS qualification pathways: An approach. (QEI Report, Institute of Educational Technology). The Open University. Available on Scholarship Exchange.
Edwards, C. (2021). Qualification pathways analysis of BA (Honours) Health and Social Care, R26. (QEI Report, Institute of Educational Technology). The Open University. Available on Scholarship Exchange.
Edwards, C., & Gaved, M. (2020a). The student study experience – analysing student study choices. In 29th EDEN (European Distance Education Network) Annual Conference 2020 - Human and Artificial Intelligence for the Society of the Future, 21-24 Jun 2020, Politehnica University, Timisoara, Romania. ORO: http://oro.open.ac.uk/70779/.
Edwards, C., & Gaved, M. (2020b). Understanding student experience: A pathways model. In L@S ‘20: Proceedings of the Seventh ACM Conference on Learning @ Scale, Virtual Event USA, 12-14 Aug 2020 (pp. 265–268). Association for Computing Machinery. Available at http://oro.open.ac.uk/70886/.
Edwards, C., & Gaved, M. (2019a). BA (Honours) Language Studies, Q30: An exploration of student study paths. (QEI Report, 2019, no.3 Institute of Educational Technology). The Open University. Available on Scholarship Exchange.
Edwards, C. & Gaved, M. (2019b). Relationship between study gaps and overlap in Stage 1 of Q07 Psychology. (QEI Report, 2019, no.1 Institute of Educational Technology). The Open University. Available on Scholarship Exchange.
Herodotou, C., Maguire, C., Mcdowell, N. D., Hlosta, M., & Boroowa, A. (2021). The engagement of university teachers with predictive learning analytics. Computers & Education, 104285.
Herodotou, C., Naydenova, G., Boroowa, A., Gilmour, A., & Rienties, B. (2020a). How can predictive learning analytics and motivational interventions increase student retention and enhance administrative support in distance education?. Journal of Learning Analytics, 7(2), 72-83.
Herodotou, C., Rienties, B., Hlosta, M., Boroowa, A., Mangafa, C., & Zdrahal, Z. (2020b). The scalable implementation of predictive learning analytics at a distance learning university: Insights from a longitudinal case study. Internet and Higher Education (IHE), 45, 100725
Herodotou, C., Muirhead, D. K., Aristeidou, M., Hole, M. J., Kelley, S., Scanlon, E., & Duffy, M. (2020c). Blended and online learning: A comparative study of virtual microscopy in Higher Education. Interactive Learning Environments, 28(6), 713–728. Available at http://oro.open.ac.uk/56427/.
Herodotou, C., Rienties, B., Boroowa, A., Zdrahal, Z., & Hlosta, M. (2019). A large-scale implementation of predictive learning analytics in higher education: The teachers’ role and perspective. Educational Technology Research and Development, 67(5), 1273–1306.
Kubiak, C., & Ullmann, T. D. (2020). Student support advisors: context, practice and impact. (QEI Report, Institute of Educational Technology). The Open University. Available on Scholarship Exchange.
Rets, I., Herodotou, C., Bayer, V., Hlosta, M., & Rienties, B. (2021). Exploring critical factors of the perceived usefulness of a learning analytics dashboard for distance university students. International Journal of Educational Technology in Higher Education, 18, article 46.
Ullmann, T.D. (2019). Automated analysis of reflection in writing: Validating Machine Learning Approaches. International Journal of Artificial Intelligence in Education, 29(2), 217-257.
Ullmann, T., & Rienties, B. (2021). Using text analytics to understand open-ended student comments at scale: Insights from four case studies. In M. Shah, J.T.E. Richardson, A. Pabel, & B. Oliver (Eds.), Assessing and enhancing student experience in higher education (pp. 211-233). Springer International Publishing. doi:10.1007/978-3-030-80889-1_9.
Ullmann, T.D. (2021a). Exploring differences in the student experience of ethnic minorities: Insights from text analytics of student comments. (QEI Report, Institute of Educational Technology). The Open University. Available on Scholarship Exchange.
Ullmann, T.D. (2021b). Supporting student academic skills development - an evaluation of an English for Academic Purposes pilot: Quantitative evaluation. (QEI Report, Institute of Educational Technology). The Open University. Available on Scholarship Exchange.
Ullmann, T.D., Hidalgo, R., Van Ameijde, J., & Murphy, Y. (2021). SEaM text analytics for the Analytics for Action programme: A first small-scale exploration. (QEI Report, Institute of Educational Technology). The Open University. Available on Scholarship Exchange.
Ullmann, T.D. (2020). Propensity score analysis of the L161 peer assessment activity. (QEI Report, Institute of Educational Technology). The Open University.
Ullmann, T.D., & Brasher, A. (2020). Text analytics to inform module changes: TASC workshop report. (QEI Report, Institute of Educational Technology). The Open University. Available on Scholarship Exchange.